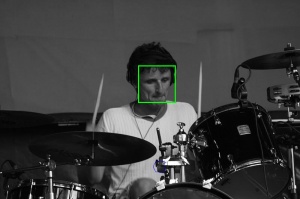
Probabilistic pattern classifiers can be used according to a frequentist or a Bayesian approach. Image courtesy of Wikipedia
Obtaining labeled data is expensive and difficult. So how can researchers use weakly labeled data? Join us for a seminar by guest speaker Dr. Dmitry Vetrov, titled: «LEARNING FROM INCOMPLETE DATA: A BAYESIAN PERSPECTIVE»
When: August 13, 2014 13.30 – 15.00
Where: Beijing-1 Auditorium, China cluster Skolkovo School of Management
SEMINAR ABSTRACT:
In his talk Dr. Dmitry Vetrov will review several research projects that are carried out in the Bayesian methods research group at Moscow State University.
Although the projects cover various areas (text processing, computer vision, machine learning) they have much in common: all rely on probabilistic models that are built from incomplete (or weakly labeled) data. Such modelling becomes very important since more and more large data-sets with weak labeling are available.
Dmitry will describe several models that are being developed in his group: latent semantic model that combines probabilistic model with Bayesian non-parametrics and deep learning; multi-label deep shape model; multi-utility segmentation model. Also on the agenda: several theoretical directions of Dr. Vetrov’s work on applying tensor methods for working with multi-dimensional distributions.
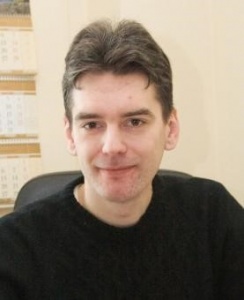
Dr. Dmitry Vetrov
SPEAKER INTRODUCTION:
Dmitry Vetrov has graduated from Moscow State University in 2003 and got his PhD in cybernetics in 2006. Currently he is leading Bayesian methods research group in Moscow State University. In 2014 he became the head of the department of big data and information retrieval on the newly created faculty of computer science in Moscow Higher School of Economics. The area of his research interests includes machine learning, graphical models, computer vision, Baysian modeling. He is (co)author of more than 120 papers including top-rank journals and conferences.