Prof. Ivan Oseledets the leader of the Skoltech Scientific Computing Group and his PhD student Evgeny Frolov have proposed recommender system, which recommendations are sensitive to both positive and negative users feedback. Their work have been accepted to ACM RecSys 2016 conference. This is a prestigious annual international conference on recommender systems. This year it will take place in Boston in September. Preprint can be found in Arxiv.
Recommender systems help to personalize user experience in content consumption based on an individual taste. Recommender systems are widely spread nowadays and if you are an active Internet user, it is hard not to see them in action. For example, when you visit an online page of your favorite bookselling store you are likely to get a personalized reading list based on your previous purchase history and personal preferences. Netflix, Amazon, Pandora, Spotify and many other companies generate a considerable amount of revenue with recommendations they produce. Broadly speaking, recommender systems can be applied in many areas for almost any kind of goods. You no longer have to seek for advice from your friends and family when choosing which movie to watch tonight, which book to read next or which hotel to book during your vacation days. Recommender systems might find an answer to this kind of questions in a blink of an eye.
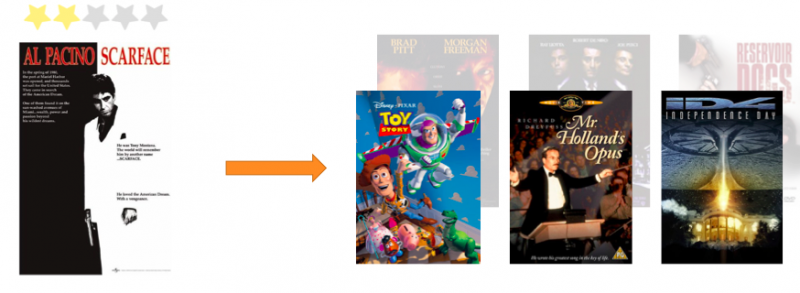
«If a user gives a low rating to the “Scarface” movie (which has a criminal narrative and contains violence), our system will find movies with “opposite” features.», E. Frolov.
Many modern recommender systems require a long history of previous user interactions to be collected before a valid recommendations model is built. But what if you are a new visitor on a web site and your preferences are yet to be discovered? This problem is known as a cold-start. Typically, this problem is solved by preparing a non-personalized list of the most representative items that is shown to a user at first. User is then expected to select (or rate) a certain number of the most interesting items from that list. Potential issue with this approach is that the list might not resonate well with actual user tastes which makes it hard to collect sufficient number of positive feedback, required to generate relevant recommendations. Such a situation is poorly handled by conventional recommendation algorithms. Given information only about the products you don’t like the system is likely to generate irrelevant recommendations.
In contrast, the model proposed by the scientists is equally sensitive to any type of user feedback. “If a user gives a low rating to the “Scarface” movie (which has a criminal narrative and contains violence), standard algorithms will tend to suggest similar (criminal) movies such as the “Godfather”. However, our system in that case will find movies with “opposite” features. For example, it could be a romance or an animation. With our algorithm there are generally no restrictions on the amount of positive feedback that must be provided by a user. Even recommendations produced from a single negative feedback will make sense. This opens up a perspective of engaging with the user instantly, right after the first feedback and without long questionnaires. It alleviates the problem of cold-start and makes experience more personalized and smooth. And of course, the more user interacts with the system, the more accurate are recommendations generated by it,” Evgeny Frolov says.
That level of recommendations quality and sensitivity became possible due to an effective use of tensors, which are a higher order generalization of matrices. Wise selection of computational techniques has made the algorithm applicable for real-time applications, where delay between user actions and system’s response is required to be minimal. The proposed model may find its applications in different areas, such as online shops or media content providers.
* The Skolkovo Institute of Science and Technology (Skoltech) is a private graduate research university in Skolkovo, Russia, a suburb of Moscow. Established in 2011 in collaboration with MIT, Skoltech educates global leaders in innovation, advances scientific knowledge, and fosters new technologies to address critical issues facing Russia and the world. Applying international research and educational models, the university integrates the best Russian scientific traditions with twenty-first century entrepreneurship and innovation.
Contact information:
Skoltech Communications
+7 (495) 280 14 81