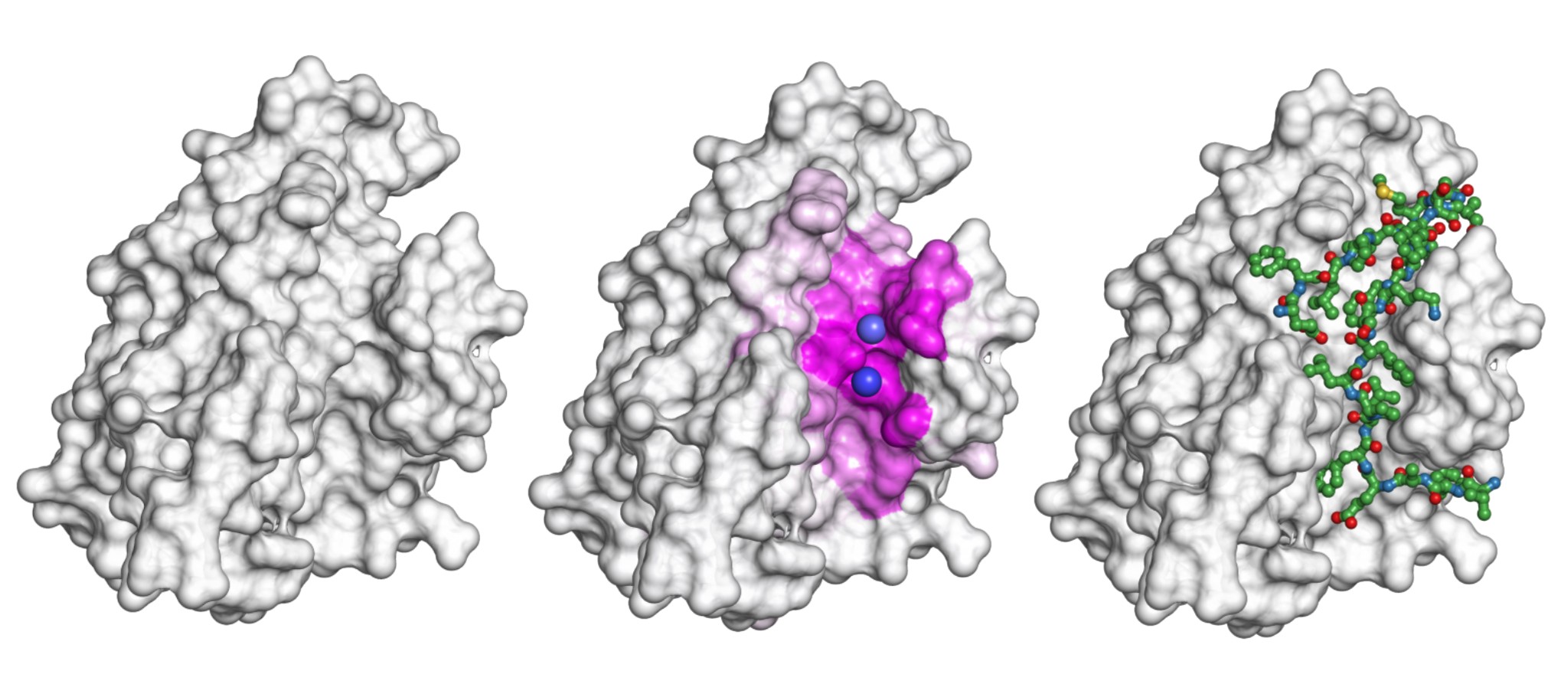
The gray shape is a protein. For the scenario of this protein binding to the peptide shown as a greenish stick-and-ball model on the right, the model presented in the study highlights the surface involved in the interaction (the pink area in the middle) and predicts the exact binding sites (purple spheres). Credit: Igor Kozlovskii and Petr Popov / Skoltech
Two Skoltech researchers have presented a highly efficient neural network model that uses data on the structure of proteins to predict which of their parts interact with other biological molecules called peptides. Knowing this is useful for developing drugs based on peptides, which can affect protein-protein interactions within cells in a targeted and nontoxic way, regulating a wide range of cellular processes. The study came out in the Journal of Chemical Information and Modeling.
Proteins are the machinery of cells, moving about, engaging with each other, and running all sorts of operations. Pharmacologists have always been intrigued by the prospect of tinkering with the interactions between proteins. Yet they seemed to be off-limits as a potential drug target: The larger therapeutic molecules, called biologics, could not penetrate into the cell to act on proteins, while small-molecule agents often proved incapable of such action.
Peptides, which naturally mediate or regulate about 40% of cellular processes, occupy a promising middle ground and hold prospects for medications targeting protein-protein interactions. Peptides offer the best of both worlds: Like small molecules, they can penetrate the cell membrane to actually reach their targets, and they also exhibit low toxicity, along with high affinity and specificity (strong and focused action) — the hallmarks of biologics.
To design peptide-based drugs, pharmacologists need to know the so-called binding sites for any given protein target. That is, the spots on the protein that can bind to a peptide. The more such sites are known, the more opportunities for drug design are available.
Researchers can identify binding sites experimentally, for example, using X-ray crystallography, which reveals the 3D structure of crystallized proteins by studying how they diffract X-rays. But this is very expensive to do for a long list of molecules, and computational methods offer a faster and cheaper alternative. Some of them draw on machine learning techniques, and as more data on the structures of protein-peptide complexes is accumulated, these methods grow more powerful and deliver ever better binding site predictions.
In their July 22 paper in the Journal of Chemical Information and Modeling, Skoltech PhD student Igor Kozlovskii and Assistant Professor Petr Popov from the iMolecule group presented a computational method called BiteNetPp, which harnesses the power of 3D convolutional neural networks to detect protein-peptide binding sites. In BiteNetPp, a known protein structure is fed to a neural network, which then highlights suspected peptide binding sites, and outputs a set of putative 3D coordinates, along with the associated probability scores.
Petr Popov comments on the approach to binding site detection as image recognition, originally introduced in the team’s earlier paper and carried over into the study reported in this story: “Just like neural networks can be trained to recognize, say, pedestrians or cyclists in ordinary 2D photos, we view binding site detection as spotting a particular kind of object in an image. The difference is we use 3D atomic structure data as our inputs, so the model operates on ‘voxels,’ a three-dimensional analogue of pixels.”
The newly presented model actually builds on the one in the previous paper. “This is called domain adaptation. BiteNetPp is the first model to have been fine-tuned on a protein-peptide dataset after initially being trained on protein-small molecule data,” Popov explains. “You can imagine this as training a model to identify places where cyclists tend to stop in the street, but you begin with data on where pedestrians tend to stop — and only then extend your domain to cyclists. Rather than start from scratch, you retrain the model, anticipating that the ‘binding sites’ for cyclists might share some similarities with the ones attracting pedestrians: you know, ice-cream stands, traffic lights, that sort of thing.”
The model’s creators have demonstrated that BiteNetPp consistently outperforms existing state-of-the-art methods by comparing their predictions for those protein-peptide binding sites that are known through experimental observations. Importantly, the new model takes less than a second to analyze a single protein structure, making it well-suited for large-scale studies. There are thousands of protein-protein interactions potentially targetable by peptide-based drugs, so computational methods have to be fast enough to make their screening feasible in a pharmacological context.
BiteNetPp is available at https://sites.skoltech.ru/imolecule/tools/bitenet/.
Contact information:
Skoltech Communications
+7 (495) 280 14 81